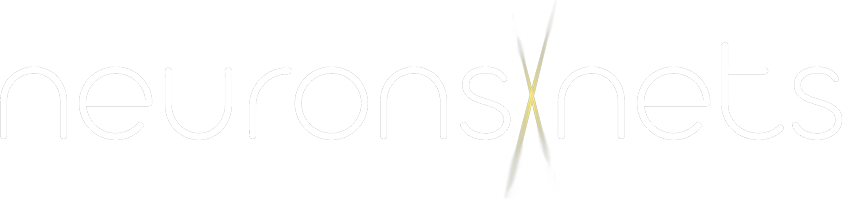
Network Analysis in Neocortex during Passive and Active Learning
What ?
How does the brain perform the complicated computations that allow us to learn about and interact with the environment? The rapid advances in new optical imaging, the powerful statistical analysis/machine-learning techniques, and the availability of computational resources, provide a unique opportunity to decipher this fundamental question. NeuronsXnets forms an international, multidisciplinary and intersectoral collaboration network involving leading groups in neuroscience, neuromorphic computing, data science, systems, optical tools/imaging, and hospitals. It takes advantage of this unique environment to improve the understanding of neural circuit function and integrate its findings in deep learning architectures and in neuromorphic circuits, aiming to develop a new generation of computing technologies based on the organizing principles of the biological nervous system, optimized for higher levels of cognition.
Project Objectives
-
Objective 1
Sub-Network Identification and Characterization in Neo-Cortex -
Objective 2
Transfer of Information between Layers in Neo-Cortex;
Network Architectures in Neo-Cortex -
Objective 3
Study how Learning Alters Cortical Network Architecture in V1 -
Objective 4
Study how Learning alters Cortical Network Architecture in PFC -
Objective 5
Network Analysis at Multiple Spatio-temporal Scales -
Objective 6
Develop scalable platforms for efficient analysis and data sharing -
Objective 7
Develop a deep-learning architecture based on the identified neural dynamics -
Objective 8
Develop advanced neuromorphic platforms and analog VLSI for fast emulations of identified functional networks -
Objective 9
Improvements in two-photon imaging techniques: (1) increase the number of neurons that can be imaged and the depth of the layers in the cortex and (2) increase the sensitivity (e.g. detection of smaller events)
Societal Challenges
Developing new methods and tools for neural circuit analysis will have broad applicability to understanding neural circuit function in various normal and disease states. For example, the proposed methodology and analysis could be also applied on data obtained from mice with various conditions (neurological disorders), such as epilepsy. Despite decades of cellular research and the discovery of over 100 genes for epilepsy in humans and mouse models, a critical gap in knowledge that needs to be bridged is to understand in detail how this highly correlated state of firing arises at the neuronal circuit level.
Partners
Brain-Inspired Computing
Brain-Inspired Computing Wednesday, May 11th, 2022, 16.00-17.00GR Dr. Angeliki Pantazi IBM Research-Zurich Biological neural networks are equipped with unique temporal dynamics and an inherent capability to continuously adapt through online learning, both enabling them to perform intelligent tasks unattainable yet by today’s AI systems. Next-generation computing technologies focus on power- and area-efficient architectures and learning ... Read more
neuronsXnets Kickoff meeting
MSCA H2020 – MSCA-RISE – 2020 Network Analysis in Neocortex during Passive and Active Learning Kickoff Meeting on Tuesday, September 28th, 2021 via zoom Join Zoom Meeting: https://us02web.zoom.us/j/88198266607?pwd=cU9FVXQxREszTldJTnhRemtoTGNIUT09 Meeting ID: 881 9826 6607 Passcode: 069950 The meeting will be recorded; Local Administration: Giannis Gavalas, FORTH, gavalas@ics.forth.gr Session 1. Introduction 12.00 GR – 12.30 GR Brief ... Read more